As cars become more autonomous, it will be critical that they know their own position to a high degree of accuracy, so they can navigate and make driving decisions effectively.
Today, for example, human drivers have the job of ensuring the car is in the appropriate lane, and switching lanes if it isn’t. But self-driving and driverless vehicles will need to be able to do this kind of verification and decision-making themselves.
That requires a step change in precision from today’s in-vehicle sat-nav systems. Those systems rely on GPS receiver equipment that generally calculates a position accurate to tens of metres. That’s fine for working out roughly how far it is to the next junction, but not for selecting the right lane at exactly the right time.
Sensor fusion is key to high-quality positioning, navigation and timing
What’s needed for autonomous vehicles is a positioning and navigation solution that’s accurate, reliable, continuous, resilient and precise to the centimetre level. Anything less would pose an unacceptable safety risk.
Autonomous vehicle developers are exploring different approaches to achieving that level of quality, but all involve combining data from a mix of sensors such as GPS/GNSS, lidar, radar, sonar, cameras, RTK, WiFi, cellular, and inertial measurement units like accelerometers and gyroscopes.
The challenge lies in testing the efficacy and reliability of those sensors. Developers need to understand the performance of each individual sensor in real-world driving conditions, as well as the reliability of the sensor fusion algorithms that process the data to enable continuous navigation.
Real-world testing isn’t sufficient or practical
While testers need to understand real-world performance, it’s not possible to do this solely by driving the vehicle in the real world. In a 2016 paper entitled Driving to Safety, Rand Corporation calculated that a self-driving car would have to drive hundreds of millions – and sometimes hundreds of billions of miles – on real-world roads for testers to be certain about its safety levels; an undertaking that would require tens or even hundreds of years.
As the authors note: “Developers and testers cannot drive their way to safety. Our findings support the need for alternative methods to supplement real-world testing in order to assess autonomous vehicle safety.”
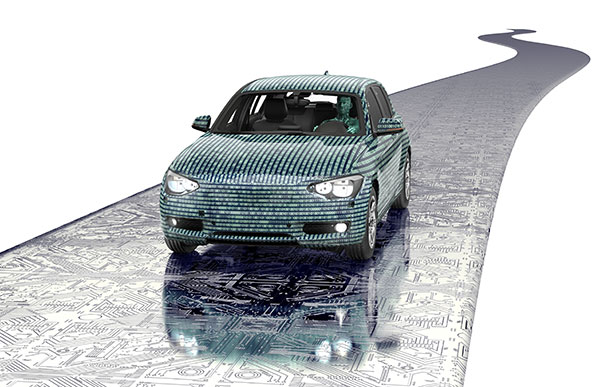
Simulation with hardware in the loop
One of those alternative methods is lab simulation with hardware in the loop. By testing physical hardware – individual sensors, or a whole driveable car model – in a simulated driving environment, developers can quickly answer questions like: if bright light blinds the lidar, can the vehicle still calculate a precise position? What about if rain or dirt gets on the camera, or multipath signals confuse the GNSS receiver? Or if all three happen at once?
Simulation is the only viable way to understand the vehicle’s behaviour in specific scenarios that may not present themselves often in the real world – or that are too risky to test in reality. What if the car comes to a pedestrian crossing in low light and a person is about to cross the road? Will the vehicle “see” the person and brake, or will it continue, potentially causing an accident?
The only way to test every conceivable scenario is to use simulation. And simulation also has other advantages: it’s faster and cheaper, and it allows developers to replay scenarios exactly – or to make controlled changes to them – to verify the impact of any changes made to the vehicle control system.
Hardware in the loop simulation at Warwick University
A simulation environment involves the integration of many different hardware and software elements to create a comprehensive test bench. At Spirent, we are working with CAV research and test labs around the world to help researchers create robust test beds for the GNSS-derived positioning, navigation and timing capabilities of highly automated and autonomous vehicles.
Working with the Intelligent Vehicles research facility at Warwick University, we are also developing a WiFi signal simulator, which can be used in conjunction with GNSS simulators to test the fusion of GNSS and WiFi position data, and to understand the efficacy of WiFi signals for positioning in areas where GNSS may be unavailable, like underground car parks and tunnels.
Find out more about PNT testing with Spirent
As the automotive industry edges closer to putting highly-automated and autonomous vehicles on our roads, only the most comprehensive testing of their position, navigation and timing capabilities will allow them to do so safely. To learn more about how Spirent can help, visit spirent.com/pnt or contact Spirent to discuss your simulation requirements.