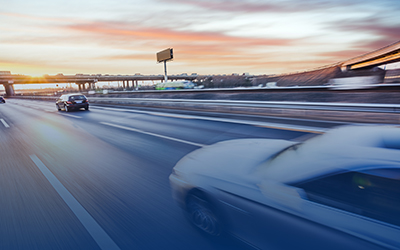
The self-driving cars of the near future will always need to know where precisely they are on the road. Only by knowing their exact position will they be able to stay in the right lane and negotiate features like junctions and roundabouts without endangering other road users.
Developers of automated driver-assist systems (ADAS) and fully autonomous control systems are exploring different ways of obtaining reliable sub-centimetre positioning accuracy. While specific approaches vary, the solution generally lies in taking data from multiple sensors on the vehicle and applying an algorithm to synthesise the data in real time into a continuous, accurate position.
Global navigation satellite systems (GNSS) like GPS, Glonass, BeiDou and Galileo are one such sensor. They play a key role in providing a vehicle with an absolute position on the earth’s surface, but despite recent advances, the accuracy of that position remains at the tens of metres level.
On its own, GNSS is not yet capable of providing the continuous, reliable, centimetre-level accuracy that autonomous vehicles need.
RTK and PPP increase GNSS accuracy to centimetre level
However, two technologies are capable of refining the accuracy of GNSS signals to centimetre and decimetre level respectively: Real-Time Kinematic (RTK) and Precise Point Positioning (PPP).
Both solutions rely on ground-based reference stations that use additional data from navigation satellites, plus advanced error correction algorithms, to generate a much more accurate signal. They then transmit this signal to the GNSS receiver in the autonomous vehicle.
RTK systems use a ground-based reference station at a fixed, known location to process and transmit the error-corrected signal to a receiver in a moving vehicle. It is generally accurate to the centimetre level, and works in real time, but each reference station only has a range of 10-20km.
PPP uses a global network of ground stations to generate an accurate signal, and transmits it to subscribers via the internet or geostationary satellite. It is accurate to the tens of centimetres, but the receiver in the vehicle needs 20-60 minutes to align with the PPP signal before it can rely on it.
Both technologies are already proven and well established in industries where highly automated or fully autonomous vehicles need to know their precise position in order to carry out their intended function. These include mining, construction and precision agriculture.
Thorough testing will be essential for autonomous vehicles to be safe
Self-driving cars, however, present more advanced challenges for RTK and PPP systems. The range over which a car is likely to drive means that one RTK base station won’t be sufficient to cover every journey. The time required to converge with the PPP signal means the in-vehicle system will need to employ workarounds like minimising the need for cold starts, and using multi-frequency receivers to reduce the convergence time.
Then there’s the continuity of the signal. If anything affects the base station’s or the vehicle’s ability to receive a signal (whether the GNSS signal or the error-corrected signal), the vehicle may be temporarily unable to use RTK or PPP to compute its precise location. If that happens, its ability to rely on data from other sensors, and to recover swiftly from the error state, will be critical.
Finally, in comparison with mining, construction and agriculture, which take place in restricted, unpopulated and/or highly controlled areas, the stakes are much higher for autonomous vehicles that may be sharing a public highway with other human road users.
All of this means that developers must conduct rigorous and professional testing of RTK and/or PPP as integral elements of a system that will also use many other technologies for positioning – from lidar and radar to cameras, inertial sensors and cellular networks.
Real-world testing is inadequate: simulation is required
Testing such advanced systems purely in the real world is impossible. It’s generally accepted that autonomous vehicles need to be test-driven over hundreds of millions of miles of varied terrain in order to expose them to the range of scenarios they may be expected to encounter in real life. That would take decades, even if suitable test tracks were available. Additionally, testers have little control over real-world conditions, and it’s difficult to repeat a real-world scenario in its full detail.
Simulation is the obvious answer, but it has to model the real world, in all of its richness, as realistically as possible. Researchers will want to simulate GNSS signals with RTK and PPP error correction messages as realistically as possible, in a huge range of scenarios.
Advanced simulation technology will be essential
To do that, researchers will need advanced signal simulators, capable of emulating all GNSS frequencies and constellations, together with RTK and PPP correction messages, in any conditions – including in the presence of multipath, signal obscuration and RF interference.
Spirent has both the technology and the expertise to enable RTK and PPP simulation for connected and autonomous vehicles. Drawing on our experience of testing precision receivers for mining, construction and agriculture, we can help you to understand the performance of RTK and PPP as elements of an overall positioning system.
Find out more
Click here to learn more about how Spirent can help, or contact Spirent directly to discuss your simulation requirements.